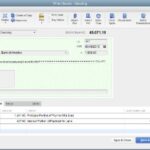
Data leaders also must consider the implications of security risks with the new technology—and be prepared to move quickly in response to regulations. With machine learning technologies, computers can be taught to analyze data, identify hidden patterns, make classifications, and predict future outcomes. The learning comes from these systems’ ability to improve their accuracy over time, with or without direct human supervision. Machine learning typically requires technical experts who can prepare data sets, select the right algorithms, and interpret the output. As market pressures to adopt AI increase, CIOs of financial institutions are being expected to deliver initiatives sooner rather than later.
More broadly, gen AI could transform compliance and security measures, enabling firms to meet regulatory requirements more efficiently while reducing the cost and effort involved in combating financial fraud and managing risk. Learn about Deloitte’s offerings, people, and culture as a global provider of audit, assurance, consulting, financial advisory, risk advisory, tax, and related services. Computer vision is the ability of computers to identify objects, scenes, and activities in a single image or a sequence of events. The technology analyzes digital images and videos to create classification or high-level descriptions that can be used for decision-making. Business units that do their own thing on gen AI run the risk of lacking the knowledge and best practices that can come from a more centralized approach. They can also have difficulty going deep enough on a single gen AI project to achieve a significant breakthrough.
Appendix: The AI technology portfolio12
Making the right investments in this emerging tech could deliver strategic advantage and massive dividends. Explore the main themes that emerged in the results, including data issues and recruitment of AI experts. Learn how the c-suite views the best tax software of 2021 for the self the AI capabilities of their company compared to the developers building the applications.
Deloitte Insights Podcasts
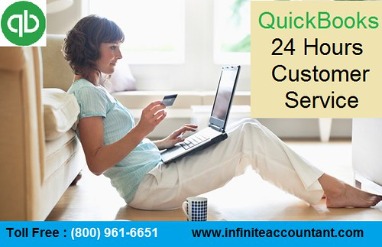
Financial institutions that successfully use gen AI have made a concerted push to come up with a fitting, tailored operating model that accounts for the new technology’s nuances and risks, rather than trying to incorporate gen AI into an existing operating model. We have observed that the majority of financial institutions making the most of gen AI are using a more centrally led operating model for the technology, even if other parts of the enterprise are more decentralized. A financial institution can draw insights from the details explored in this article, decide how much to centralize the various components of its gen AI operating model, and tailor its approach to its own structure and culture. An organization, for instance, could use a centralized approach for risk, technology architecture, and partnership choices, while going with a more federated design for strategic decision making and execution. Gen AI, along with its boost to productivity, also presents new risks (see sidebar “A unique set of risks”). Risk management for gen AI remains in the early stages for financial institutions—we have seen what does capitalizing assets mean chron com little consistency in how most are approaching the issue.
The financial services industry has entered the artificial intelligence (AI) phase of the digital marathon. The right operating model for a financial-services company’s gen AI push should both enable scaling and align with the firm’s organizational structure and culture; there is no one-size-fits-all answer. An effectively designed operating model, which can change as the institution matures, is a necessary foundation for scaling gen AI effectively. Hyper-personalization – Banks and others are leveraging AI and non-financial data to better create and target highly personalized offerings. This is shifting the paradigm in FS from a reactive service to one that is truly intuitive and responsive. It now handles two-thirds of customer service interactions and has led to a decrease in marketing spend by 25%.
- As a result, the institution is taking a more adaptive view of where to place its AI bets and how much to invest.
- He is responsible for driving the Center’s research platforms and delivering world-class research for our clients.
- A financial institution can draw insights from the details explored in this article, decide how much to centralize the various components of its gen AI operating model, and tailor its approach to its own structure and culture.
- NLP powers the voice- and text-based interface for virtual assistants and chatbots.
- It now handles two-thirds of customer service interactions and has led to a decrease in marketing spend by 25%.
A checklist of essential decisions to consider
He’s written about how financial services firms can unlock the full value of generative AI, why the FS adoption of cloud computing has been slower than envisioned and lucrative niches for fintechs moving forward. In addition to his global role, David is the co-organizer of Accenture’s FinTech Innovation Lab, a mentorship program bringing together fintech start-ups and leading financial institutions, with labs in the U.K., U.S., and Asia-Pacific. Follow him for continued coverage around how financial services firms and fintechs are embracing technology, AI and data to reinvent their operations and deliver a more personalized customer experience. The second factor is that scaling gen AI complicates an operating dynamic that had been nearly resolved for most financial institutions.
Here’s what real estate firms need to watch out for before they leverage generative AI. Companies can also look at making best-in-class and respected internal services available to external clients for commercial use. Explore the free O’Reilly ebook to learn how to get started with Presto, the open source SQL engine for data analytics.
Leading corporate and investment banks, for example, have built up expert teams of quants, modelers, translators, and others who often have AI expertise and could add gen AI skills, such as prompt engineering and database curation, to their capability set. Banks with fewer AI experts on staff will need to enhance their capabilities through some mix of training and recruiting—not provision for bad debts journal entry a small task. Financial services have made considerable progress adopting gen AI in the last two years. While there’s been a sizable focus on efficiency and cost optimization thus far, many FS CIOs are eager to deliver top line growth. To do so, they’ll need to work closely with the business to consider how gen AI can lead to new ways of working, new products and new capabilities that can help accelerate revenues. The future of AI in financial services looks bright and it will be interesting to see where firms go next.